- 22 (Registered)
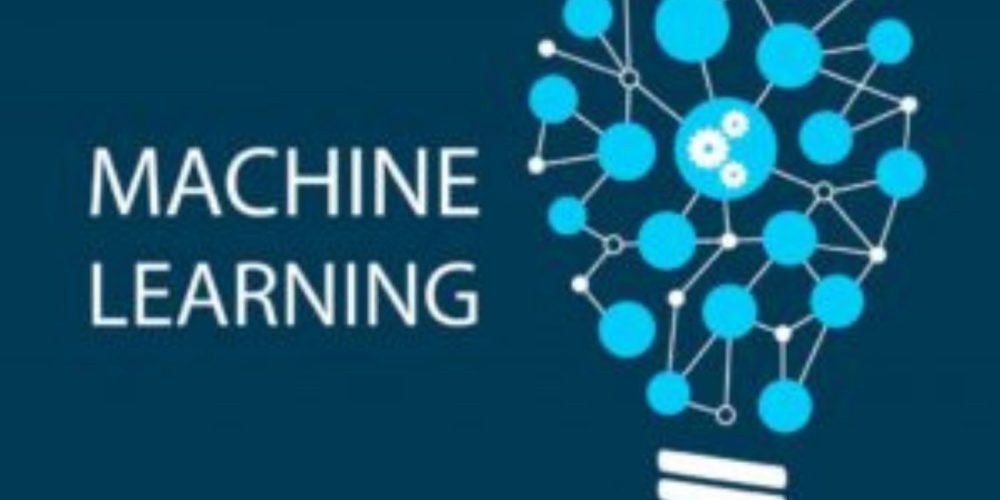
When: 4th August 2020 to 8th August 2020
Course Description
This course will introduce the learner to applied machine learning, focusing more on the techniques and methods than on the statistics behind these methods. The course will start with a discussion of how machine learning is different than descriptive statistics, and introduce the scikit learn toolkit through a tutorial. The issue of dimensionality of data will be discussed, and the task of clustering data, as well as evaluating those clusters, will be tackled. Supervised approaches for creating predictive models will be described, and learners will be able to apply the scikit learn predictive modelling methods while understanding process issues related to data generalizability (e.g. cross validation, overfitting). The course will end with a look at more advanced techniques, such as building ensembles, and practical limitations of predictive models. By the end of this course, students will be able to identify the difference between a supervised (classification) and unsupervised (clustering) technique, identify which technique they need to apply for a particular dataset and need, engineer features to meet that need, and write python code to carry out an analysis.
Course Content
-
Day 01 - Module-1 Introduction to ML 0/8
-
Lecture1.1
-
Lecture1.2
-
Lecture1.3
-
Lecture1.4
-
Lecture1.5
-
Lecture1.6
-
Lecture1.7
-
Lecture1.8
-
-
Module-2 Introduction to Python 0/4
-
Lecture2.1
-
Lecture2.2
-
Lecture2.3
-
Lecture2.4
-
-
Module-3 Data Structure & Data Manipulation 0/9
-
Lecture3.1
-
Lecture3.2
-
Lecture3.3
-
Lecture3.4
-
Lecture3.5
-
Lecture3.6
-
Lecture3.7
-
Lecture3.8
-
Lecture3.9
-
-
Module-4 Data Visualization 0/6
-
Lecture4.1
-
Lecture4.2
-
Lecture4.3
-
Lecture4.4
-
Lecture4.5
-
Lecture4.6
-
-
Day 02 - Module-1 Linear Regression - Case Study & Project 0/7
-
Lecture5.1
-
Lecture5.2
-
Lecture5.3
-
Lecture5.4
-
Lecture5.5
-
Lecture5.6
-
Lecture5.7
-
-
Module-2 Linear Regression - 2 0/3
-
Lecture6.1
-
Lecture6.2
-
Lecture6.3
-
-
Module-3 Logistic Regression 0/13
-
Lecture7.1
-
Lecture7.2
-
Lecture7.3
-
Lecture7.4
-
Lecture7.5
-
Lecture7.6
-
Lecture7.7
-
Lecture7.8
-
Lecture7.9
-
Lecture7.10
-
Lecture7.11
-
Lecture7.12
-
Lecture7.13
-
-
Day 03 - Module-1 Decision Trees 0/4
-
Lecture8.1
-
Lecture8.2
-
Lecture8.3
-
Lecture8.4
-
-
Module-2 Random Forests 0/2
-
Lecture9.1
-
Lecture9.2
-
-
Module-3 KNN 0/3
-
Lecture10.1
-
Lecture10.2
-
Lecture10.3
-
-
Module-4 Bagging and Boosting 0/5
-
Lecture11.1
-
Lecture11.2
-
Lecture11.3
-
Lecture11.4
-
Lecture11.5
-
-
Day 04 - Module-1 Naïve Bayes Algorithm 0/8
-
Lecture12.1
-
Lecture12.2
-
Lecture12.3
-
Quiz12.1
-
Quiz12.2
-
Quiz12.3
-
Quiz12.4
-
Quiz12.5
-
-
Module-2 Support Vector Machine 0/4
-
Lecture13.1
-
Lecture13.2
-
Lecture13.3
-
Lecture13.4
-
-
Module-3 Recommendation Systems 0/2
-
Lecture14.1
-
Lecture14.2
-
-
Day 05 - Module-1 Clustering 0/5
-
Lecture15.1
-
Lecture15.2
-
Lecture15.3
-
Lecture15.4
-
Lecture15.5
-
-
Module-2 Time Series 0/9
-
Lecture16.1
-
Lecture16.2
-
Lecture16.3
-
Lecture16.4
-
Lecture16.5
-
Lecture16.6
-
Lecture16.7
-
Lecture16.8
-
Lecture16.9
-
-
Module-3 Reinforcement Learning 0/3
-
Lecture17.1
-
Lecture17.2
-
Lecture17.3
-
-
Day 06 - Module-1 Artificial Neural Networks 0/8
-
Lecture18.1
-
Lecture18.2
-
Lecture18.3
-
Lecture18.4
-
Lecture18.5
-
Lecture18.6
-
Lecture18.7
-
Lecture18.8
-
-
Module-2 Convolutional Neural Networks 0/7
-
Lecture19.1
-
Lecture19.2
-
Lecture19.3
-
Lecture19.4
-
Lecture19.5
-
Lecture19.6
-
Lecture19.7
-
-
Module-3 Recurrent and Recursive Nets 0/4
-
Lecture20.1
-
Lecture20.2
-
Lecture20.3
-
Lecture20.4
-
-
Module-4 Machine learning at scale 0/3
-
Lecture21.1
-
Lecture21.2
-
Lecture21.3
-
Instructor
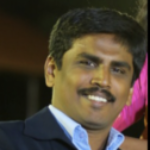
Arun Panayappan is the Founder of Techcovery Solutions which has been in the training and consulting business for three years now. Arun Panayappan was the Vice President, Platform Engineering at www.goibibo.com which is India’s largest online travel agent. He has over 23 years of IT experience including 4 years of overseas experience.Techcovery has trained over 1000 participants in Data science and mentored 4 start ups during its existence. He has worked as a consulting CTO for companies like cogknit semantics, FirstIPO and Trip38. He is frequently consulted for data science architecture and strategy by corporations across the industry. In his role at Amazon, his technical decision-making skills enabled Kindle Topaz Operations Console to go global and scale seamlessly to meet seasonal load. He drove initiatives to hire and develop the best for the Category Expansions team that doubled in size during his leadership tenure while launching 70 new stores for www.amazon.com every year. He built highly scalable web crawling systems to monitor price changes at competitor sites for millions of products sold by Amazon. This system used statistical machine learning and modeled crawl frequencies using poison distribution. His team built the on-boarding experience of vendor platform(https://vendorexpress.amazon.com) to onboard and manage small and medium size vendors to increase selection and buying options. Prior to joining Amazon, he worked for Kuliza (www.kuliza.com), young start up by ex-Amazon employees, and had served the Research team at Accenture Technology Labs., India. He is an innovative and a tech-savvy leader. He sets a high bar for himself and his team on software design, quality and processes. He has a pleasing personality and excellent communication skills.